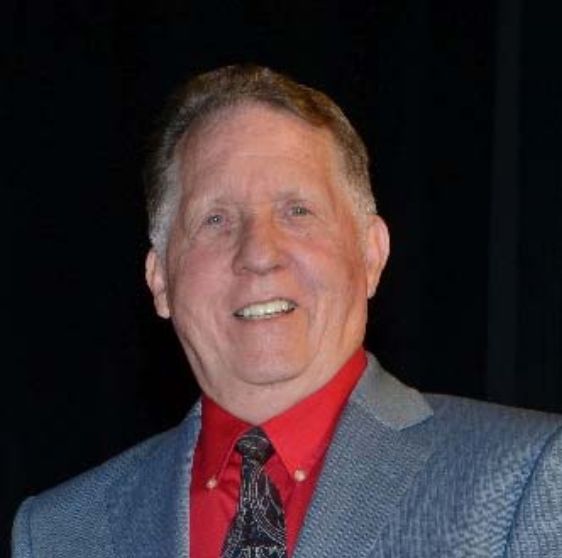
Milo Schield (Augsburg University)
Abstract
Confounding is the elephant in the intro statistics classroom. Confounding is used to show that association isn't causation -- and then it disappears. Confounding isn't mentioned in the index of most statistics textbooks. But most students in introductory statistics deal with social statistics, observational studies and big data. For them confounding is a much bigger influence than random sampling error. This workshop argues that we need to offer a confounder-based statistical literacy course alongside the traditional statistical inference course. This workshop introduces the topics involved in a confounder-based statistical literacy course: crude associations, confounding, mechanisms, effect size, the Cornfield conditions, study design, hypothetical thinking about plausible confounders, conditional probability using ordinary English (percent, percentage and likely grammars), multivariate regression (standardization) without needing computer software, and the influence of confounding on statistical significance. It also presents MATH 1300: a confounder-based statistical literacy course offered this fall at the University of New Mexico. This course has less than a 30% overlap with the traditional inferential statistics course. Designed for students in non-quantitative and observational majors. The audience for this workshop is statistical educators who want to provide more value for their students by offering an alternative to statistical inference. No pre-requisite or technology required. Audience interaction via periodic chat, surveys and a statistical literacy assessment. Decide whether confounder-based statistical literacy is the next big thing in statistical education.