Thursday, March 21st, 202412:00 pm – 12:30 pm ET
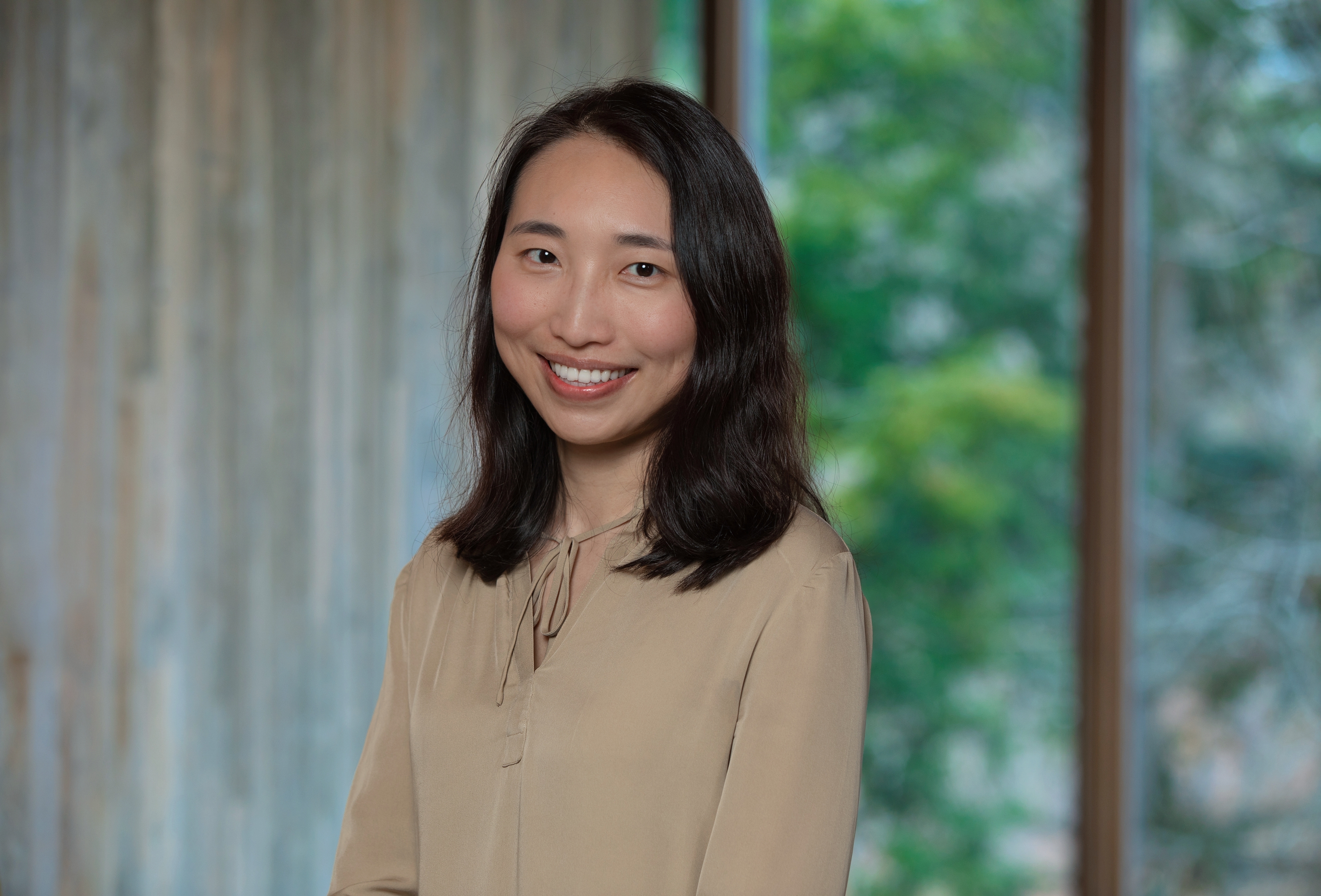
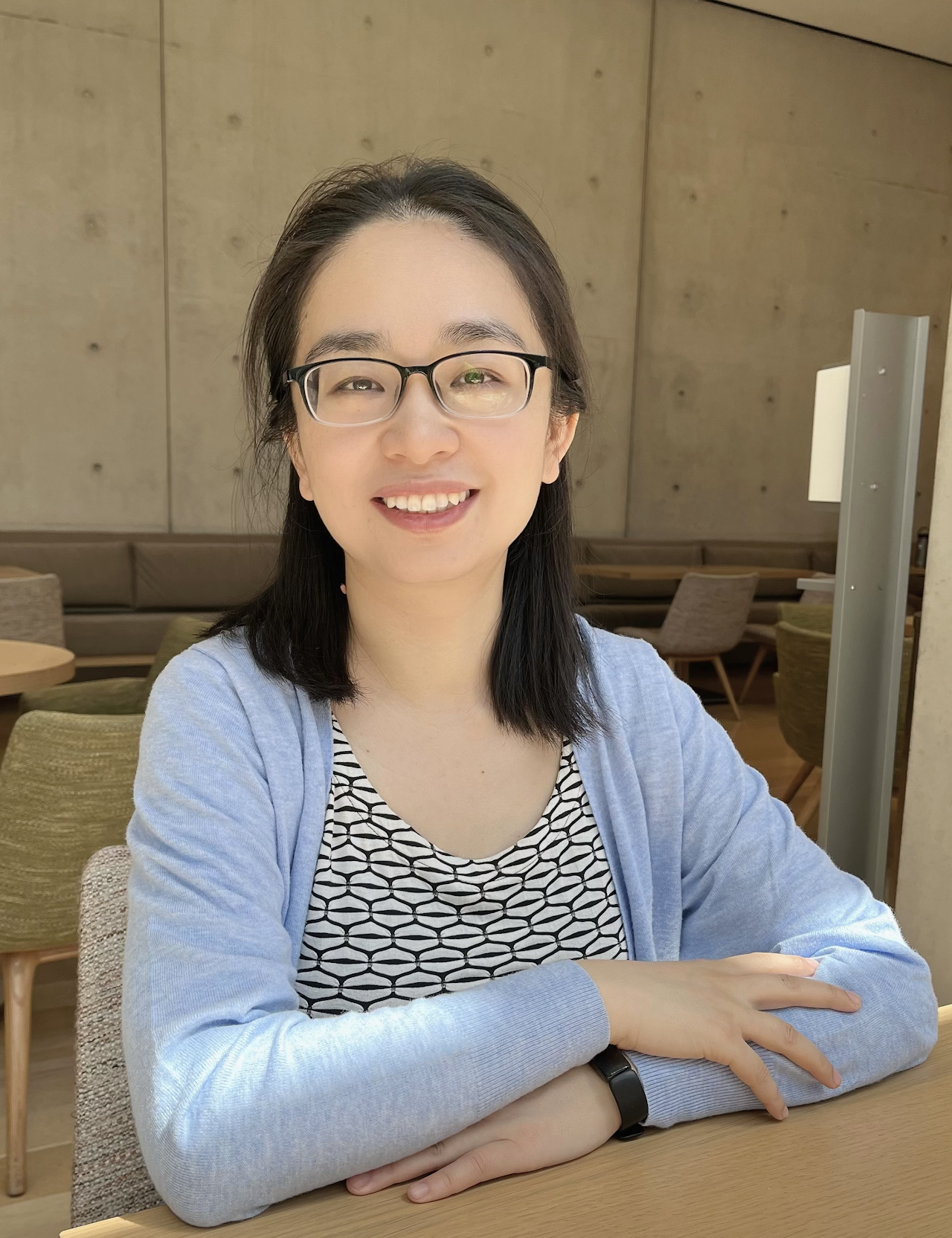
Presented by: Qing Wang (Wellesley College) and Xizhen Cai (Williams College)
Abstract
In this March edition of the JSDSE/CAUSE webinar series, we highlight the 2023 article: Active-Learning Class Activities and Shiny Applications for Teaching Support Vector Classifiers. The authors will discuss support vector classifiers, one of the most popular linear classification techniques for binary classification. Different from some commonly seen model fitting criteria in statistics, such as the ordinary least squares criterion and the maximum likelihood method, its algorithm depends on an optimization problem under constraints, which is unconventional to many students in a second or third course in statistics or data science. As a result, this topic is often not as intuitive to students as some of the more traditional statistical modeling tools. In order to facilitate students’ mastery of the topic and promote active learning, the authors have developed some in-class activities and their accompanying Shiny applications for teaching support vector classifiers. The designed course materials aim at engaging students through group work and solidifying students’ understanding of the algorithm via hands-on explorations. The Shiny applications offer interactive demonstration of the changes of the components of a support vector classifier when altering its determining parameters.